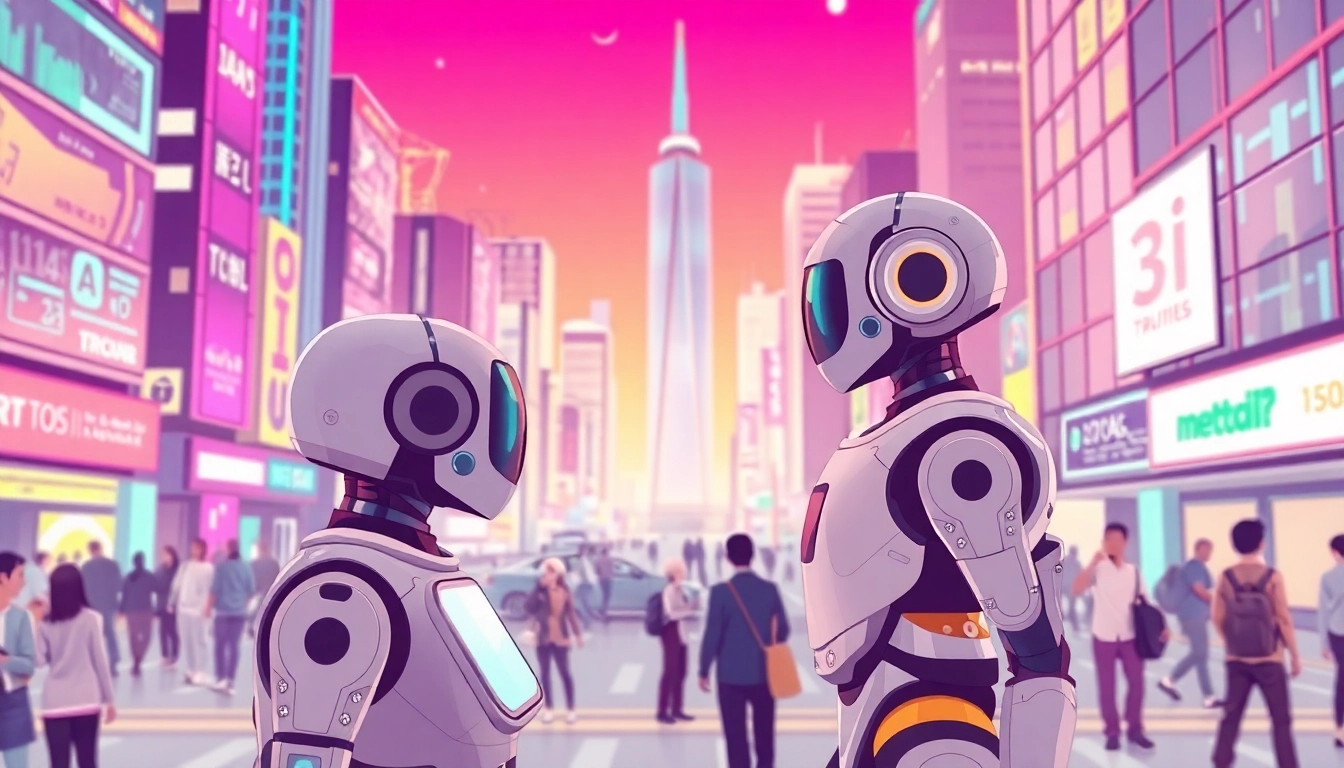
What is Agentic AI?
Definition and Overview
Agentic AI refers to a novel class of artificial intelligence systems specifically designed to operate autonomously, making decisions and taking actions based on predefined goals without the need for constant human oversight. This autonomous decision-making capability distinguishes Agentic AI from traditional AI, which often requires human input for operation. According to a study by IBM, Agentic AI systems are capable of pursuing complex tasks and can adapt their strategies in response to changing circumstances, thus demonstrating a high level of autonomy in their operations.
Key Characteristics of Agentic AI
Agentic AI is characterized by several key features that enhance its functionality:
- Autonomy: These systems can perform tasks and make decisions without human intervention, responding to immediate situations with minimal or no guidance.
- Adaptability: Agentic AI systems can learn from new data and experiences, allowing them to refine their decision-making processes and strategies over time.
- Multi-step reasoning: They can solve complex problems that require a sequence of interrelated decisions, which is essential for handling real-world tasks.
- Integration capabilities: Agentic AI systems can interface with other systems and data sources, allowing for comprehensive problem-solving across various domains.
- Scalability: Their design allows them to operate at scale, handling multiple tasks simultaneously without degradation in performance.
Comparison with Traditional AI Models
Traditional AI models typically operate under supervised settings, heavily dependent on human inputs and pre-defined algorithms. In contrast, Agentic AI embodies a paradigm shift where the system itself is capable of autonomous decision-making.
For instance, traditional machine learning approaches often require substantial labeled data for training, whereas Agentic AI can learn more efficiently using minimal data through reinforcement learning, simulating decision-making in environments where human oversight is impractical. This distinction in operation highlights the enhanced capabilities and broader applications of Agentic AI across various industries.
Core Components of Agentic AI
Machine Learning Algorithms
Machine learning underpins the foundational capabilities of Agentic AI. Various algorithms, such as neural networks, decision trees, and ensemble methods, are utilized to ensure that these systems can learn and enhance their performance over time. In Agentic AI, the focus often lies on:
- Supervised Learning: Uses labeled data to train models that make predictions or decisions based on new inputs.
- Unsupervised Learning: Detects patterns and relationships within data without prior labeling, fostering greater insights autonomously.
- Reinforcement Learning: Employs a trial-and-error approach, allowing systems to develop optimal action strategies through rewards and penalties.
The adaptability and efficiency of these algorithms are critical in enabling Agentic AI to evolve and respond effectively to dynamic environments.
Decision-Making Frameworks
Integral to the functioning of Agentic AI are sophisticated decision-making frameworks such as Markov Decision Processes (MDPs) and game theory. These frameworks facilitate:
- Strategic Decision-Making: Allowing the AI to evaluate different strategies based on potential outcomes and associated risks, essential for complex problem-solving.
- Optimal Policy Development: Enabling the establishment of rules or strategies that maximize the reward over time, installing a robust decision-making heightening the effectiveness of the agent.
These frameworks empower Agentic AI systems to navigate uncertainties effectively, further enhancing their reliability in context-sensitive applications.
Real-time Adaptability
Real-time adaptability is another crucial component of Agentic AI. These systems are equipped to adjust not only their actions but also their internal models based on real-time feedback from their environments. This can include:
- Continuous Learning: The ability to update its knowledge repository as new data becomes available.
- Context-awareness: Understanding and adapting to the surrounding context, which is essential for effective decision-making in changeable environments.
Such adaptability is increasingly imperative in sectors like healthcare and finance, where rapid changes in data can significantly impact outcomes.
Applications of Agentic AI
In Business Operations
Agentic AI significantly transforms business operations by automating processes and enhancing decision-making efficiency. Examples include:
- Supply Chain Management: AI agents autonomously analyze data from various sources to optimize inventory levels, predict demand, and identify delivery challenges in real-time.
- Customer Service: Through conversational agents and chatbots, businesses deploy Agentic AI to handle inquiries autonomously, significantly reducing wait times and improving customer satisfaction.
- Marketing Automation: AI analyzes market trends and consumer behaviors to devise and execute targeted marketing campaigns without manual oversight.
In Healthcare Innovations
The healthcare industry has seen substantial contributions from Agentic AI, particularly in enhancing diagnostic accuracy and operational efficiency. Applications include:
- Predictive Analytics: AI systems forecast patient outcomes based on historical data, helping to personalize treatments and improve patient care.
- Telemedicine: Agentic AI supports remote monitoring and diagnosis, enabling healthcare providers to manage patients proactively.
- Drug Discovery: AI algorithms streamline the identification of potential drug candidates and predict their effectiveness through autonomous simulations.
In Autonomous Vehicles
In the automotive industry, Agentic AI plays a crucial role in developing self-driving vehicles. Key functionalities include:
- Environmental Perception: Utilizing sensors and cameras, AI agents analyze their surroundings and respond to changing conditions, making real-time driving decisions.
- Path Planning: AI systems determine the safest and most efficient routes while adapting to road conditions and traffic laws dynamically.
- Risk Assessment: Autonomously evaluating potential hazards and dynamically altering driving strategies to mitigate risk.
These advancements are pushing the boundaries of technology, promising safer and more efficient transportation solutions.
Challenges and Ethical Considerations
Autonomy vs. Control
One of the most pressing issues surrounding Agentic AI is the balance between autonomy and human control. While the advantage of autonomy is evident, it raises concerns regarding accountability and the quality of decisions made without human oversight. Key considerations include:
- Responsibility: Determining who is liable for decisions made by AI—whether it’s the developers, organizations, or the AI itself.
- Decision Transparency: Variances in AI decision-making processes lead to a demand for clear explanations of how decisions are reached.
Bias and Fairness in AI Systems
Another significant concern is bias in AI systems, which can result from skewed training data leading to unfair outcomes. Organizations must actively work to:
- Assess and Mitigate Bias: Regularly review data sets and AI results to identify and minimize biases.
- Implement Fairness Guidelines: Establish frameworks for ethical AI usage and decision-making, promoting diversity and fairness in outcomes.
Regulatory and Compliance Issues
As AI continues to evolve, regulatory frameworks must adapt to address the unique challenges presented by Agentic AI. This includes ensuring compliance with data protection laws, such as GDPR, and developing governance standards for AI technologies. Key areas of focus may include:
- Data Privacy: Ensuring that personal data is handled ethically and securely, respecting individual privacy rights.
- Accountability Protocols: Establishing governance structures to oversee AI use and address compliance breaches effectively.
The Future of Agentic AI
Trends and Predictions
The future of Agentic AI is poised for rapid growth and evolution, with significant trends affecting its development:
- Increased Integration: Expect to see wider adoption across industries, as organizations seek to leverage AI for competitive advantage.
- Human-AI Collaboration: Systems that augment human decision-making with autonomous insights will emerge, reshaping workflows and enhancing productivity.
Impact on Job Markets
The rise of Agentic AI will undoubtedly influence job markets. While some roles may diminish due to automation, new opportunities will arise in AI management, training, and oversight. Workers will need to adapt by acquiring skills that complement AI capabilities, emphasizing collaboration over competition.
Advancements in Technology and Research
Technological advances, such as quantum computing and advanced neural networks, are likely to amplify the capabilities of Agentic AI. Ongoing research towards achieving general AI may unlock even greater potential, leading to innovative applications that currently seem far-fetched.