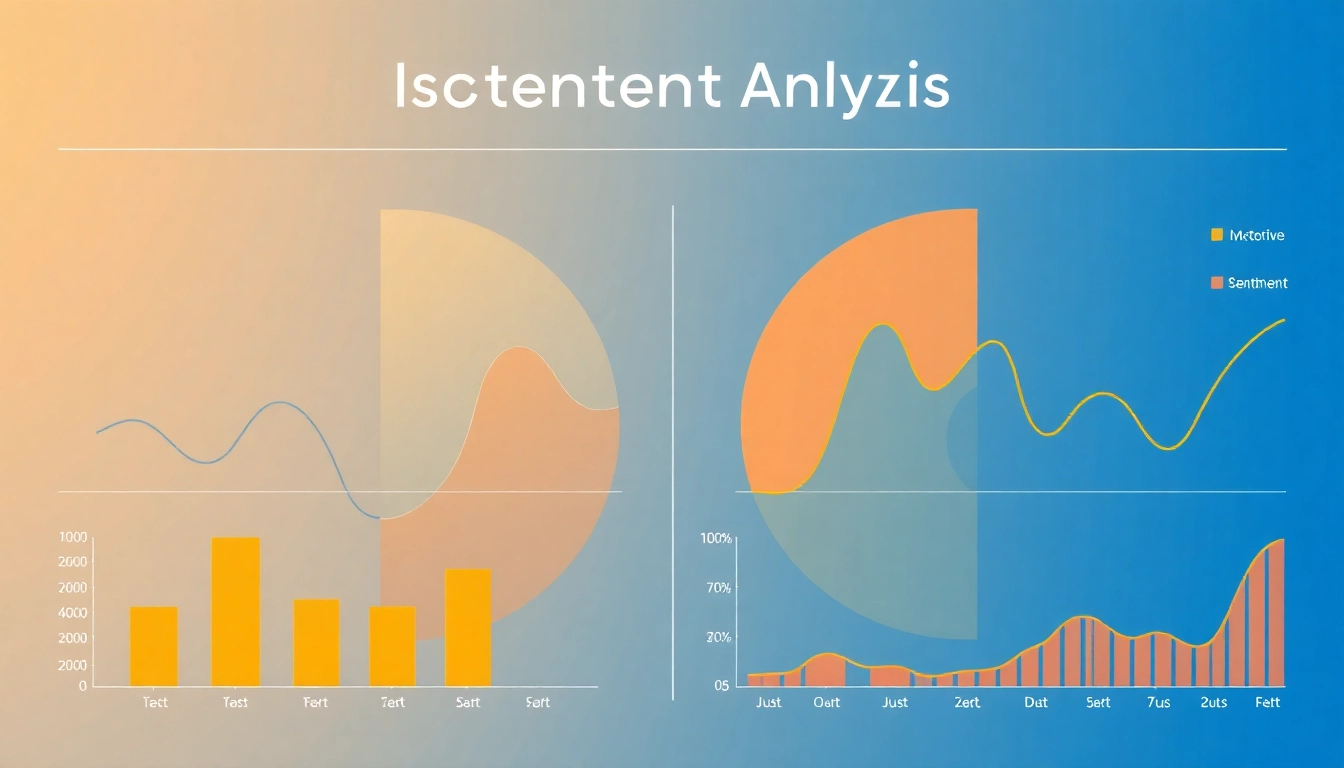
Introduction to AI Sentiment Analysis
In an era where data-driven insights are paramount, AI sentiment analysis has emerged as an indispensable tool for businesses and researchers alike. This powerful technique leverages artificial intelligence to understand and interpret the emotional tone behind textual data. Whether it’s gauging customer satisfaction through social media feedback or analyzing market sentiment towards a product launch, AI-driven sentiment analysis provides deep insights that are critical for informed decision-making.
Definition of Sentiment Analysis
Sentiment analysis, sometimes referred to as opinion mining, is the computational study of opinions, sentiments, and emotions expressed in a piece of text. This may involve analyzing products reviews, social media posts, customer feedback, or any form of communicative text. The primary aim is to categorize the sentiment portrayed—whether it is positive, negative, or neutral. For example, a positive sentiment might be communicated through favorable reviews of a product, while negative sentiment could arise from complaints about the same product.
Importance of Emotional Insight
The emotional tone in business communication plays a pivotal role in shaping customer experiences, brand perception, and market dynamics. Understanding how customers feel about a product or service allows organizations to tailor their strategies and offerings accordingly. Moreover, emotional insights derived from sentiment analysis empower marketers to devise campaigns that resonate more deeply with target audiences, thus enhancing engagement and driving loyalty.
How AI Enhances Sentiment Analysis
AI significantly enhances sentiment analysis by employing sophisticated algorithms and machine learning models capable of understanding context, tone, and nuances within language. Traditional methods of sentiment analysis often lacked accuracy due to the complexity of human language. AI, particularly through natural language processing (NLP), allows for the analysis of text data at scale, making it possible to extract valuable insights from vast data sets efficiently.
Key Components of AI Sentiment Analysis
Natural Language Processing (NLP)
At the heart of AI sentiment analysis is natural language processing (NLP), which focuses on the interaction between humans and computers using natural language. NLP technologies analyze textual data, enabling machines to comprehend, interpret, and respond to human language in a valuable way. Techniques such as tokenization, part-of-speech tagging, and named entity recognition are commonly employed to prepare data for sentiment analysis.
Machine Learning Techniques
Machine learning plays a crucial role in enhancing the capacity of sentiment analysis tools to adapt and improve over time. By using supervised learning methods, models can be trained on labeled datasets, learning to classify sentiments based on previously annotated examples. Techniques such as support vector machines, decision trees, and neural networks have gained popularity due to their effectiveness in classifying sentiment data with high accuracy.
Data Sources for Analysis
The effectiveness of sentiment analysis tools heavily depends on the quality and diversity of data used for analysis. Typical data sources include:
- Social Media Platforms: Twitter and Facebook provide a wealth of real-time data that captures public sentiment on various topics.
- Customer Reviews: Websites like Amazon and Yelp host valuable consumer opinions that can reveal customer satisfaction levels and product performance.
- Surveys and Polls: Direct feedback from customers collected through surveys can be a rich source of sentiment data.
Applications of AI Sentiment Analysis
Marketing Strategy and Brand Management
AI sentiment analysis is a game-changer in marketing strategy and brand management. By understanding consumer opinions, businesses can fine-tune their messaging, identify target demographics, and enhance brand loyalty. For instance, companies can monitor how their brand is perceived in real-time, addressing negative sentiments promptly and capitalizing on positive public opinions to reinforce their marketing campaigns.
Customer Feedback and Support
Sentiment analysis tools are invaluable for customer support teams. By analyzing incoming feedback from various channels, organizations can identify common pain points, assess satisfaction levels, and improve service quality. Moreover, sentiment analysis can automate the routing of customer inquiries based on emotional tone, ensuring that frustrated customers receive immediate assistance.
Social Media Monitoring
Social media platforms are a goldmine for understanding public sentiment about brands or products. AI sentiment analysis allows businesses to monitor brand mentions, hashtags, and conversations in real-time, enabling them to react swiftly to negative feedback or capitalize on positive discussions. This proactive approach to social media management can significantly enhance brand reputation and customer satisfaction.
Challenges in AI Sentiment Analysis
Data Quality and Bias
While AI sentiment analysis has proven effective, it is not without its challenges. Data quality is a significant concern, as inaccurate or biased data can lead to misguided insights. For instance, training models on unrepresentative data sets may result in skewed sentiments, reducing the reliability of the analysis. Organizations must ensure their data sources are diverse and representative of the populations they serve.
Contextual Understanding of Language
Language is nuanced, and understanding context is critical in sentiment analysis. Sarcasm, idioms, or cultural references can easily confuse AI models, leading to incorrect sentiment classification. As such, advancing AI capabilities to grasp context remains a primary challenge. Enthusiastic efforts are underway to improve algorithms’ and machine learning models’ understanding of sentiment in varied contexts, but this remains an area needing continuous development.
Scalability of Analysis Tools
As data volumes continue to grow, so does the challenge of scaling sentiment analysis tools. Organizations require solutions that not only handle large datasets but also offer real-time insights. The complexity increases when integrating these systems with existing IT infrastructure and ensuring seamless data flow. Developers must focus on building scalable, robust systems that can adapt to growing data demands without sacrificing performance.
Future Trends in AI Sentiment Analysis
Integration of Generative AI
The future of AI sentiment analysis will likely see deeper integration of generative AI techniques, which can create more sophisticated models capable of producing richer insights. Generative AI can assist in generating more human-like responses in customer service queries, understanding the subtleties of customer emotions in varied contexts, and predicting future trends based on historical sentiment data.
Real-Time Analysis Capabilities
As businesses strive to remain competitive in fast-paced markets, the demand for real-time sentiment analysis will continue to grow. Companies will increasingly rely on tools that can provide immediate insights from data streams to inform rapid decision-making and agile marketing strategies. The development of advanced techniques and platforms to support real-time data processing will enhance organizational responsiveness.
Personalization in Customer Interactions
With advancements in AI sentiment analysis, personalization in customer interactions will reach new heights. Businesses can tailor communications and offerings based on individual sentiment profiles created through analysis. This approach not only enhances customer satisfaction but also fosters long-term loyalty as customers feel understood and valued by brands.